TWC #9
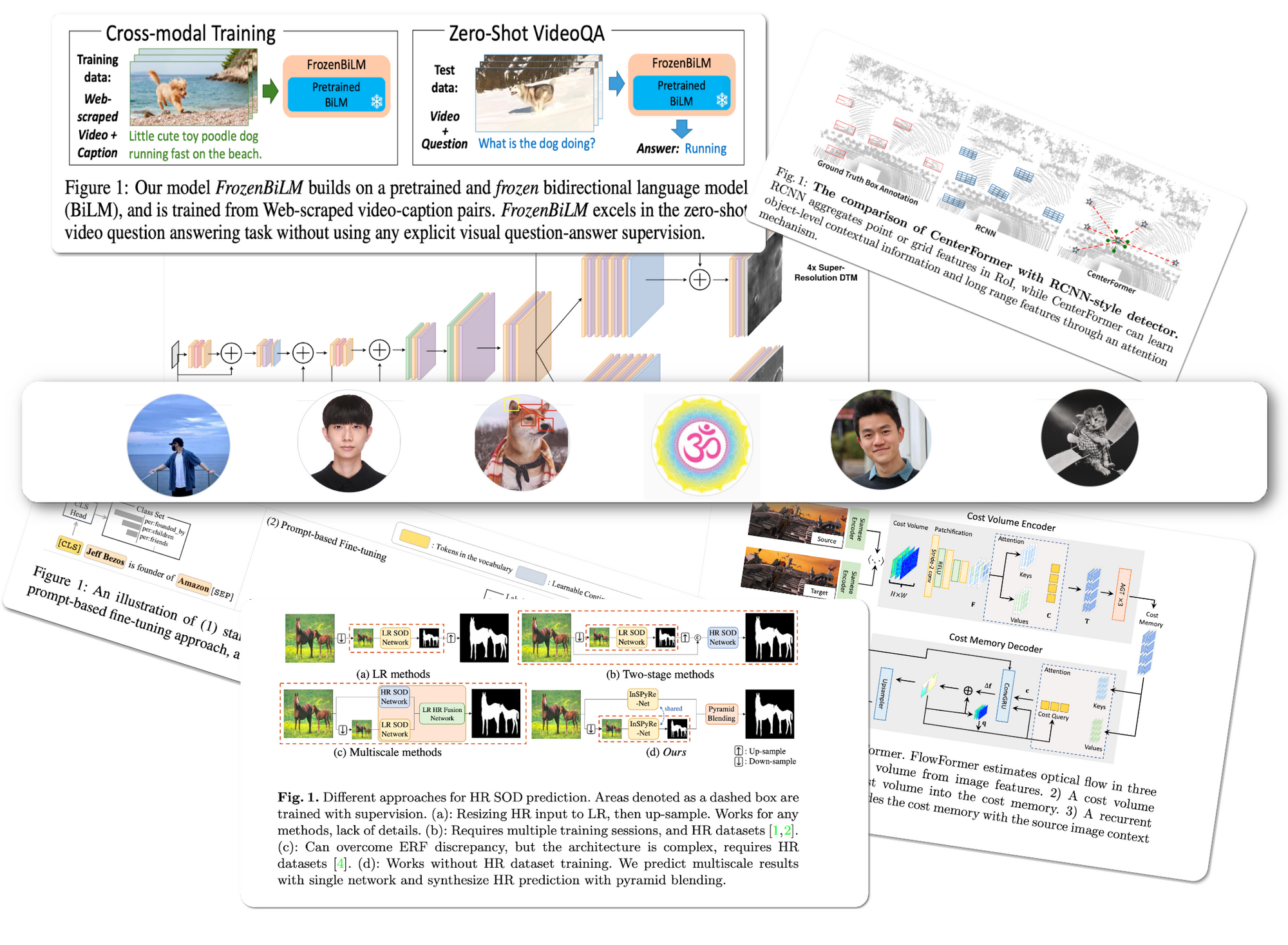
state-of-the-art (SOTA) updates for 26 Sept– 2 Oct 2022
TasksWithCode weekly newsletter highlights the work of researchers who publish their code (often with models) along with their SOTA paper. This weekly is a consolidation of daily twitter posts tracking SOTA changes.
Six papers released with code were selected for the newsletter. Four of them released models. One of them had a demo page.
To date, 27 % (86,485) of total papers (320,758) published have code released along with the papers (source)
SOTA updated last week for the following tasks
- Salient Object Detection
- Dialog Relation Extraction
- Optical Flow Estimation (Optical flow targets at estimating per-pixel correspondences between a source image and a target image, in the form of a 2D displacement field. This is used in downstream video tasks like action recognition)
- Depth estimation
- Zero-Shot Learning
- 3D Object Detection
TWC App updates
Last week we released an app to compare semantic clustering properties of embeddings. Users can compare embeddings of models from Hugging Face with GPT-3 models ranging from 350 million parameter model 175 billion parameters (pre-computed results only for LLMs due to API usage constraints). Large language model comparison has also been added to the other two embedding comparison apps - semantic similarity and semantic search.
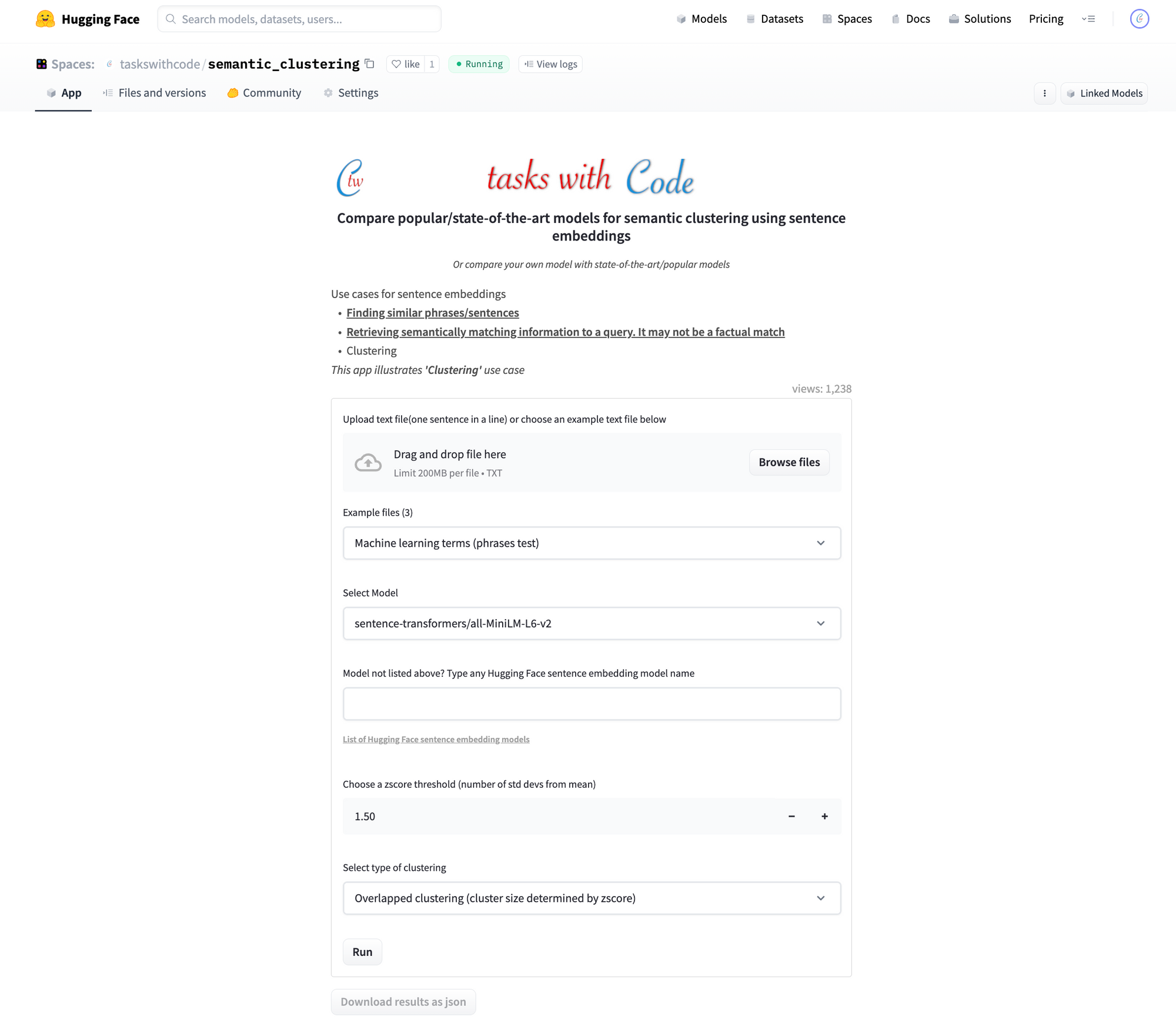
#1 in Salient Object Detection on 8 datasets
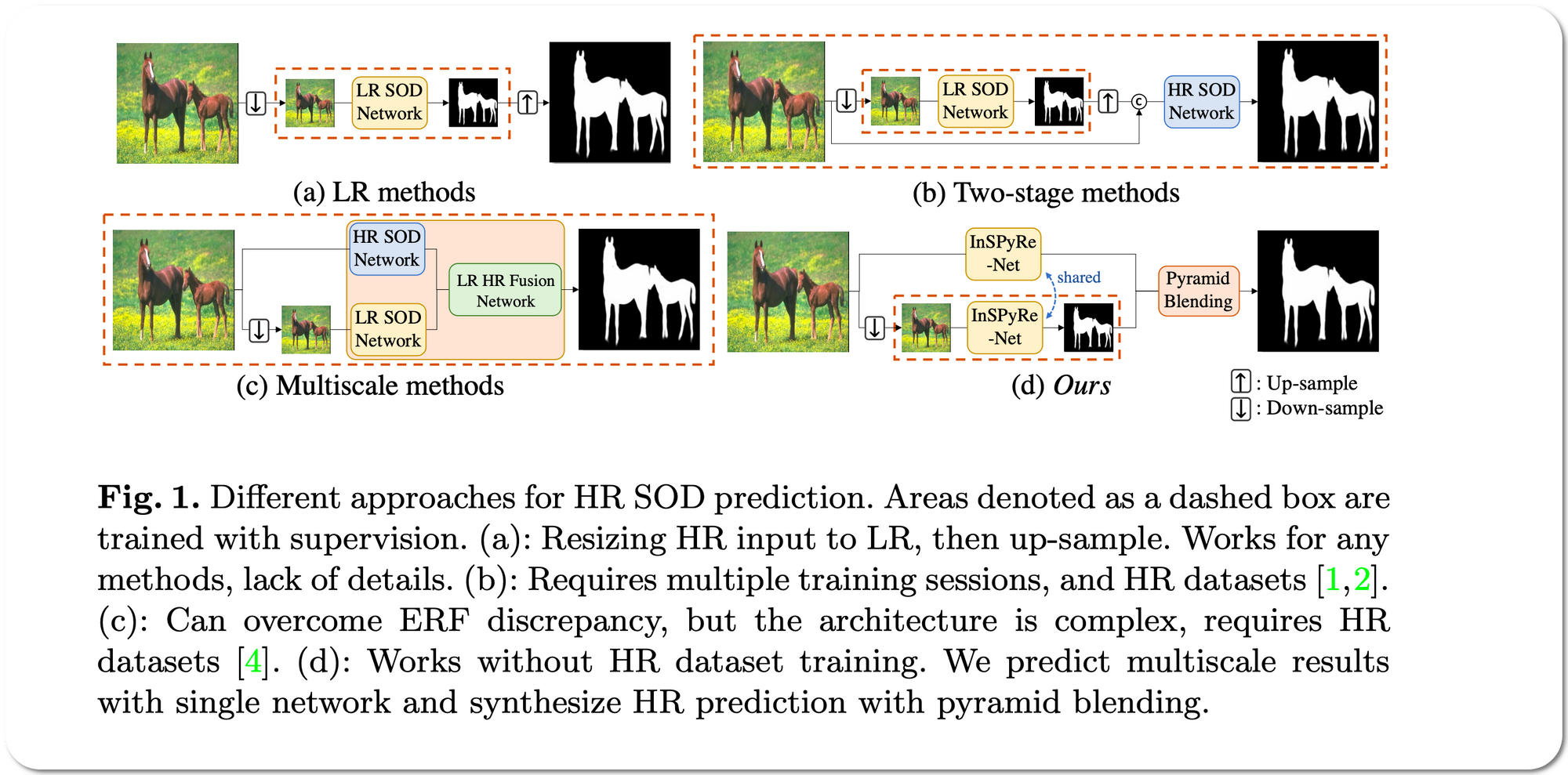
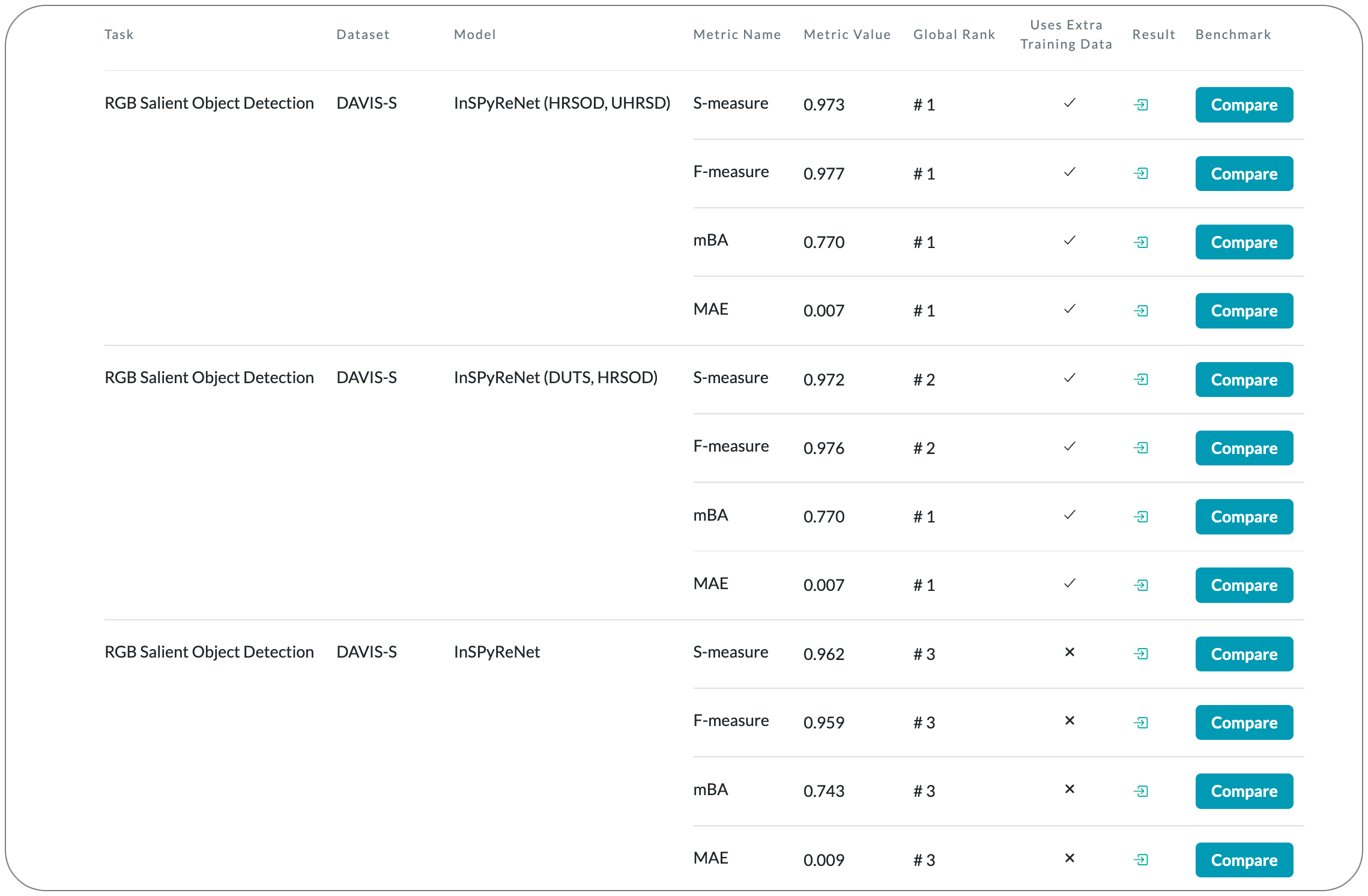

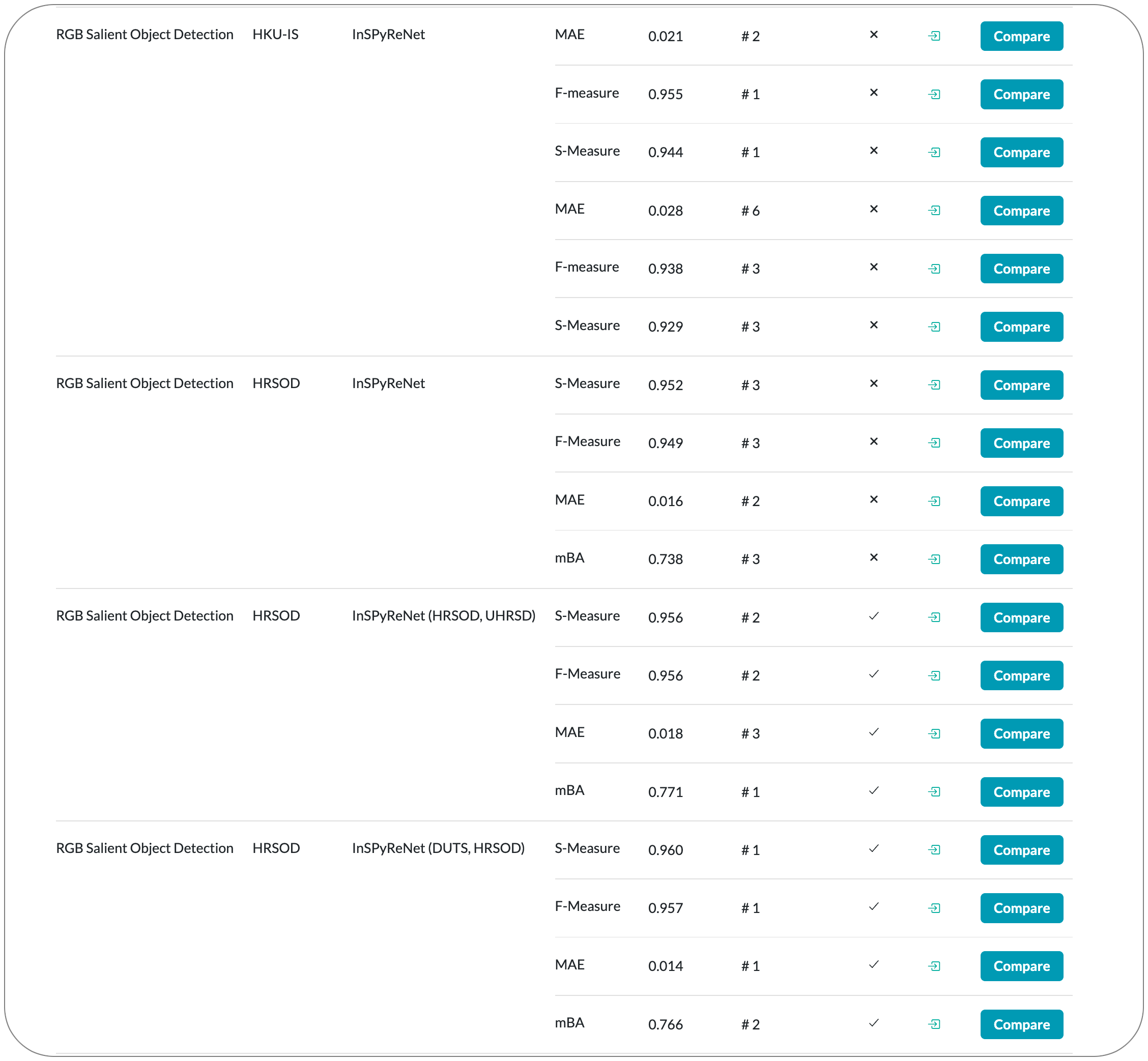


Model Name: InSPyReNet
Notes: This paper propose a model for Salient object detection (SOD) for high-resolution (HR) image prediction with out any HR dataset. Their model is designed as a image pyramid structure of saliency map, which enables to ensemble multiple results with pyramid-based image blending. For HR prediction, they design a pyramid blending method which synthesizes two different image pyramids from a pair of LR and HR scale from the same image to overcome effective receptive field (ERF) discrepancy.
Demo page link A Notebook was created to replicate inference on CPU using on one of their pretrained models. Samples shown below. Salient detection time is ~3 secs on CPU for images. For a 6 second high resolution video (1920x2080) it took 53 minutes on a CPU.
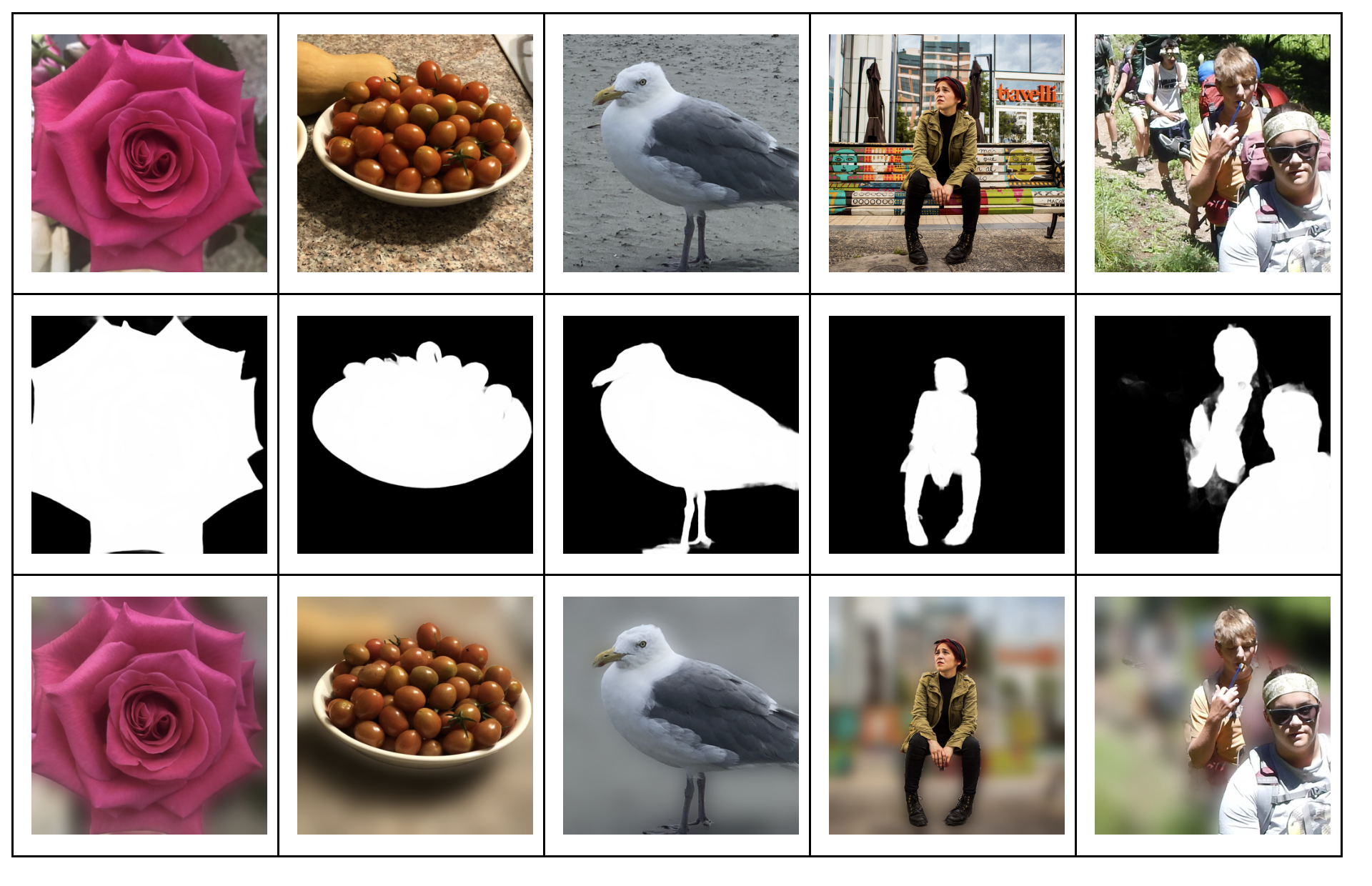
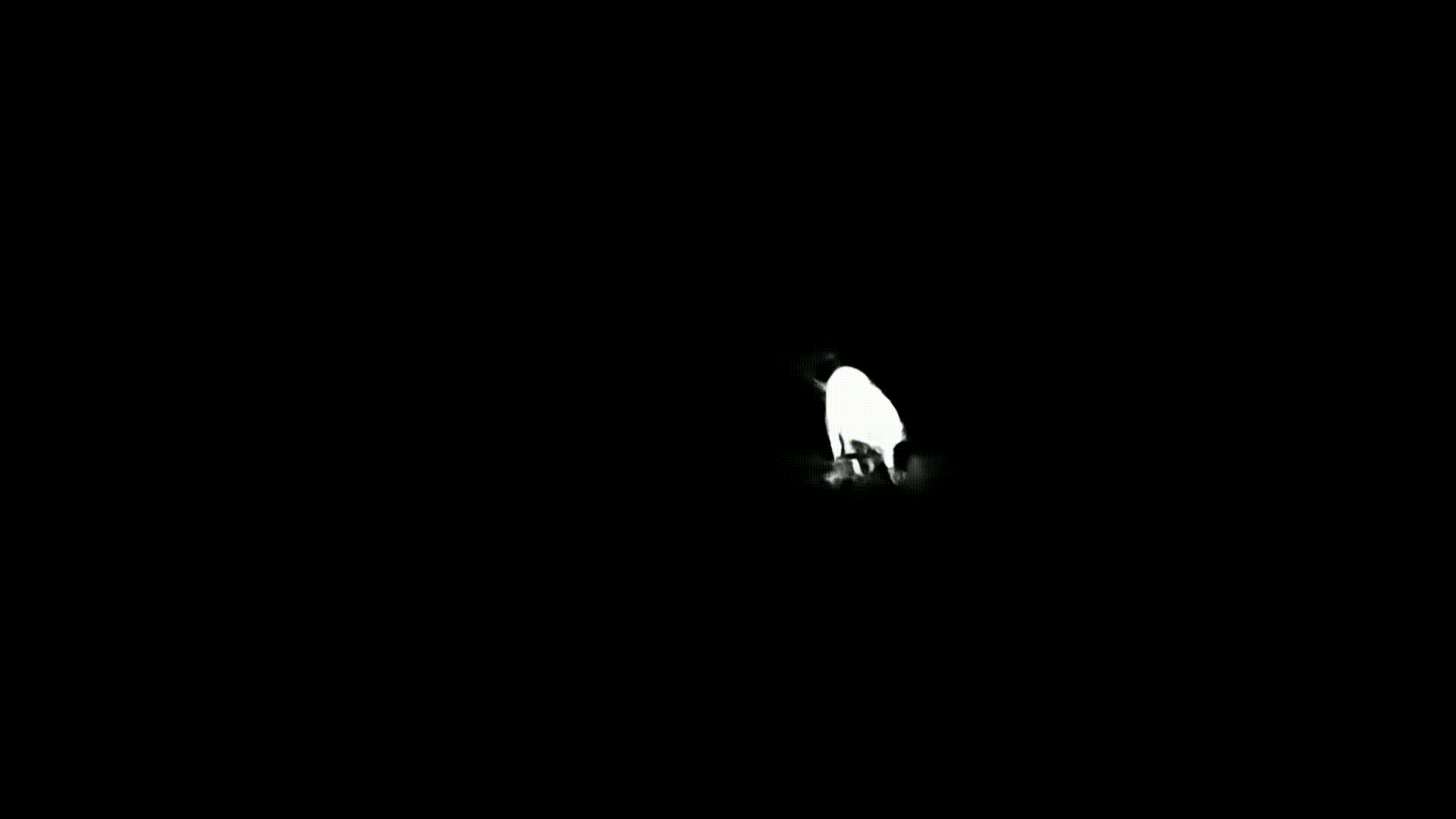
License: MIT license
#1 in Dialog Relation Extraction on 2 datasets



Model Name: GRASP
Notes: This paper aims to predict the relations between argument pairs that appear in dialogue. Most previous studies utilize fine-tuning pre-trained language models (PLMs) only with extensive features to supplement the low information density of the dialogue by multiple speakers. To effectively exploit inherent knowledge of PLMs without extra layers and consider scattered semantic cues on the relation between the arguments, this paper proposes a guiding model with relational semantics using Prompt (GRASP). They adopt a prompt-based fine-tuning approach and capture relational semantic clues of a given dialogue with 1) an argument-aware prompt marker strategy and 2) the relational clue detection task.
Demo page link None to date
License: MIT license
#1 in optical flow estimation on Sintel
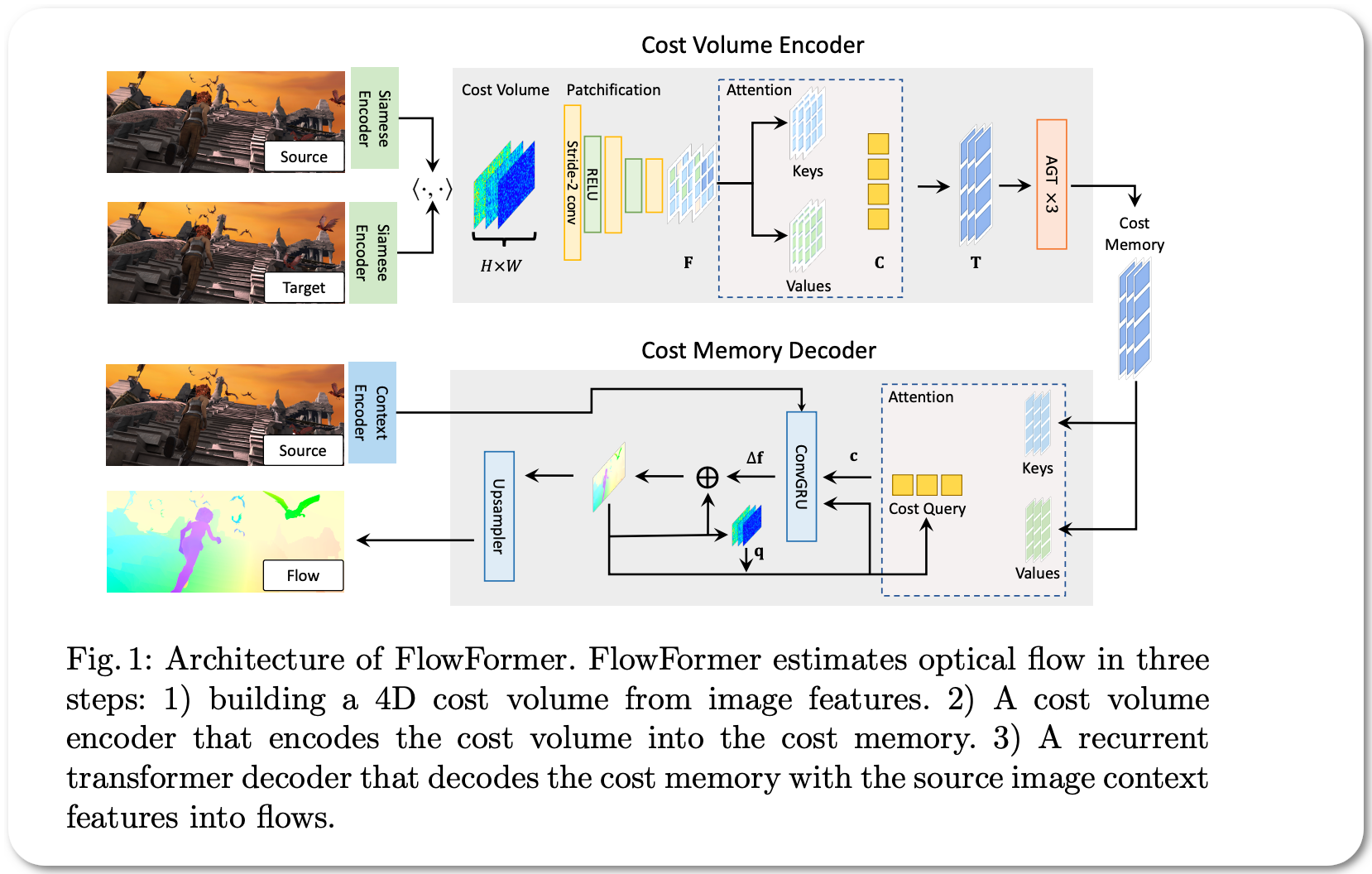


Model Name: FlowFormer
Notes: This paper introduces a transformer-based neural network architecture for learning optical flow. This model tokenizes the 4D cost volume built from an image pair, encodes the cost tokens into a cost memory with alternate-group transformer (AGT) layers in a novel latent space, and decodes the cost memory via a recurrent transformer decoder with dynamic positional cost queries.
Demo page link None to date
License: Apache license permitting commercial use
#1 in Depth Estimation on Mars DTM Estimation



Model Name: GLPDepth
Notes: This paper introduces a generative adversarial network solution that estimates the digital terrain model (DTM) at 4× resolution from a single monocular image
Demo page link Hugging Face spaces . The spaces link produces two outputs:- Image super resolution at 4x and depth estimation (the SOTA is in depth estimation - however the super resolution image is quite good qualitatively too). The input is expected to be in grayscale (RGB images converted by demo app to grayscale).
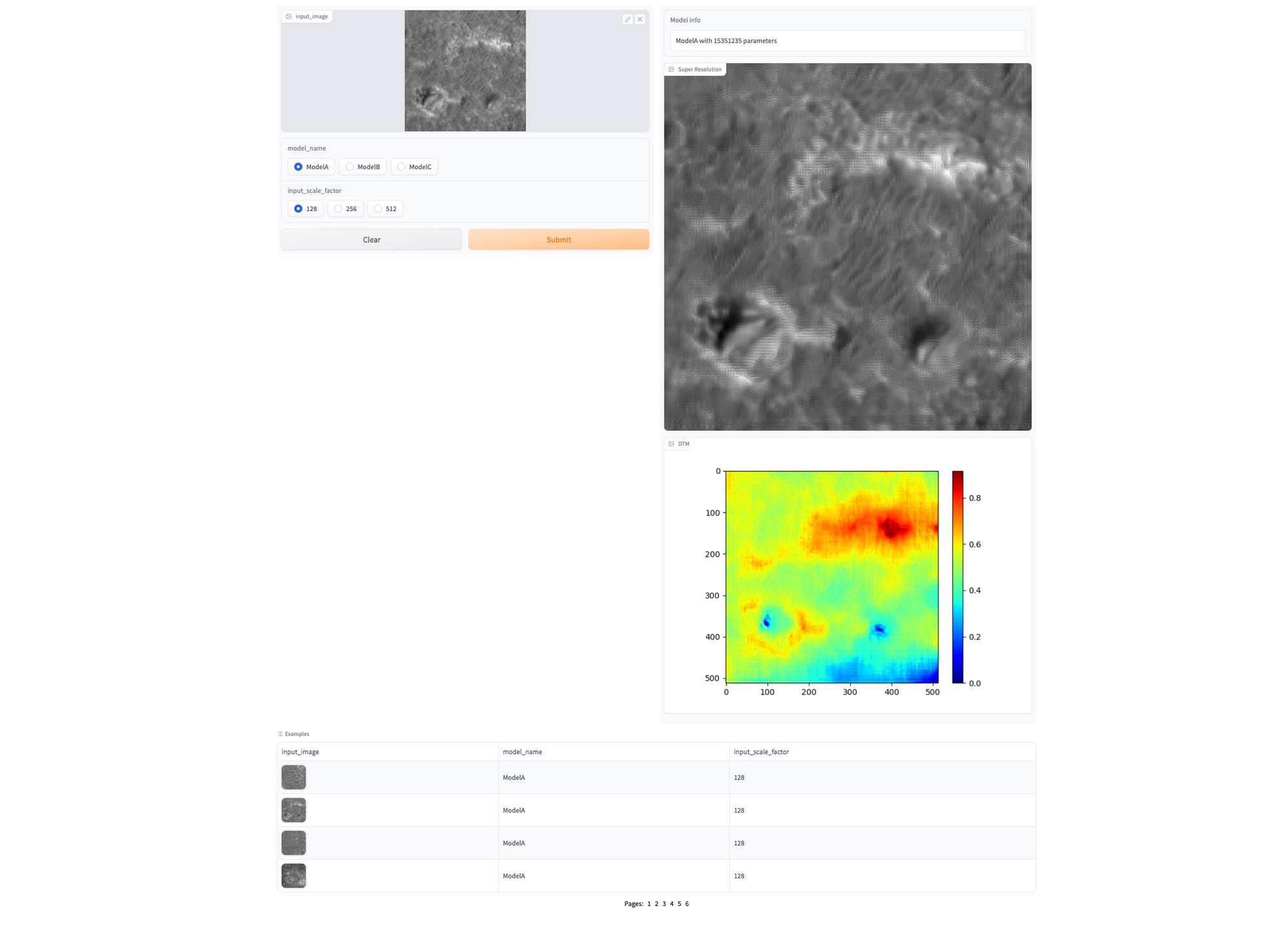
License: Not specified
#1 in Zero-Shot Learning on 8 datasets



Model Name: FrozenBiLM
Notes: This paper builds on a frozen bidirectional language models (BiLM) and show that such an approach provides a stronger and cheaper alternative for zero-shot VideoQA. In particular, (i) they combine visual inputs with the frozen BiLM using light trainable modules, (ii) and train such modules using Web-scraped multi-modal data, and finally (iii) perform zero-shot VideoQA inference through masked language modeling, where the masked text is the answer to a given question.
Demo page link None to date
License: Apache 2.0 license. Commercial use permitted following license guidelines
#1 in 3D Object Detection on Waymo dataset

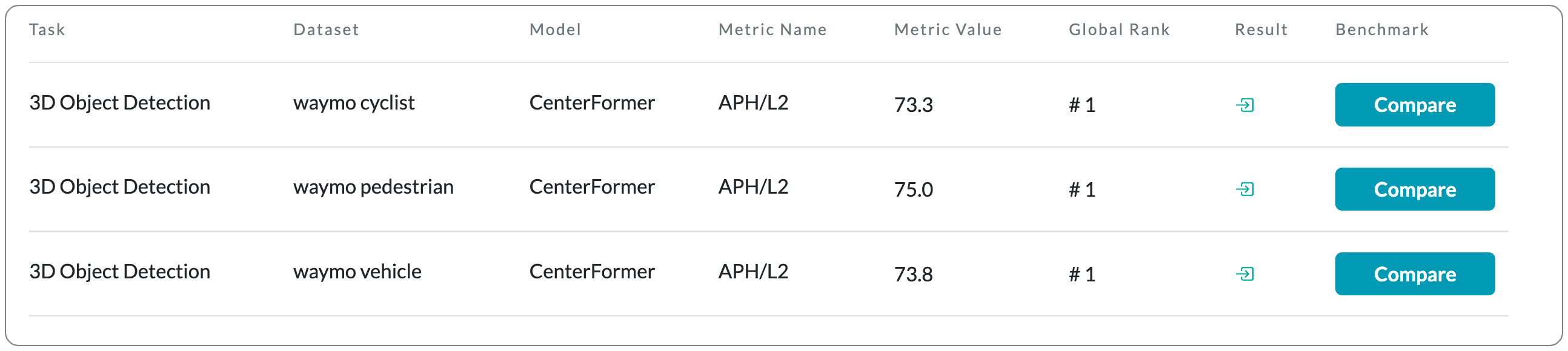

Model Name: CenterFormer
Notes: This paper proposes a center-based transformer network for LiDAR-based 3D object detection. CenterFormer first uses a center heatmap to select center candidates on top of a standard voxel-based point cloud encoder. It then uses the feature of the center candidate as the query embedding in the transformer. To further aggregate features from multiple frames, they design an approach to fuse features through cross-attention. Lastly, regression heads are added to predict the bounding box on the output center feature representation. This design reduces the convergence difficulty and computational complexity of the transformer structure.
Demo page link None to date
License: MIT license